Senior Artificial Intelligence Research Engineer. Worldcoin, Tools fo Humanity.
Development of deep neural networks for iris/face recognition at a billion-user scale.
Member of the "Artificial Intelligence and Biometrics" team.
Senior Postdoctoral Researcher. University of Cambridge, Machine Learning Group.
EPSRC grant "Machine Learning for Tomorrow" (in collaboration with Microsoft Research).
Supervisor: Richard Turner
Postdoctoral Researcher. University of Edinburgh, Machine Learning Group.
Few-shot learning with Deep Kernels in Gaussian Processes (in collaboration with Huawei).
Supervisor: Amos Storkey
Research Intern in the Camera Platform team. Snapchat (London, UK).
Disentangling latent representations in deep autoencoders.
Supervisors: Patrick-Fox Roberts, Edward Rosten
PhD in Machine Learning and Robotics. University of Plymouth (UK).
Implementing social skills in humanoid robots using machine learning techniques.
Supervisors: Angelo Cangelosi, Torbjorn Dahl, Giorgio Metta
Robotics engineer (R&D Skunkworks). Eurolink Systems group (Rome, Italy).
Autonomous control of unmanned ground/aerial vehicles (UGVs/UAVs) in critical applications such as search-and-rescue, patrolling, and bomb disposal.
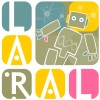
Artificial neural networks and genetic algorithms in evolutionary robotics.
Supervisor: Domenico Parisi
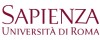
Supervisors: Stefano Puglisi Allegra, Domenico Parisi, Gianluca Baldassarre
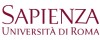
Supervisors: Marta Olivetti Belardinelli, Valerio Santangelo
Publications
Shysheya, A., Bronskill, J., Patacchiola, M., Nowozin, S., Turner, R.E. (2023). "FiT: Parameter Efficient Few-shot Transfer Learning for Personalized and Federated Image Classification". International Conference on Learning Representations (ICLR). [arXiv] [GitHub]
Patacchiola, M., Bronskill, J., Shysheya, A., Hofmann, K., Nowozin, S., Turner, R.E. (2022). "Contextual Squeeze-and-Excitation for Efficient Few-Shot Image Classification". Advances in Neural Information Processing Systems (NeurIPS). [arXiv] [GitHub]
Bronskill*, J., Massiceti*, D., Patacchiola*, M., Hofmann, K., Nowozin, S., & Turner, R.E. (2021). "Memory Efficient Meta-Learning with Large Images". Advances in Neural Information Processing Systems (NeurIPS). *Co-first authors. [arXiv]
Sendera, M., Tabor, J., Nowak, A., Bedychaj, A., Patacchiola, M., Trzcinski, T., Spurek, P., & Zieba, M. (2021). "Non-Gaussian Gaussian Processes for Few-Shot Regression". Advances in Neural Information Processing Systems (NeurIPS). [arXiv]
Patacchiola, M., & Storkey, A. (2020). "Self-Supervised Relational Reasoning for Representation Learning". Advances in Neural Information Processing Systems (NeurIPS). Spotlight (top 3%) [arXiv] [GitHub] [Poster]
Patacchiola, M., Turner, J., Crowley, E. J., O'Boyle, M., & Storkey, A. (2020). "Bayesian Meta-Learning for the Few-Shot Setting via Deep Kernels". Advances in Neural Information Processing Systems (NeurIPS). Spotlight (top 3%) [arXiv] [GitHub] [Poster]
Ochal, M., Patacchiola, M., Storkey, A., Vazquez J., & Wang S. (2021). "How Sensitive are Meta-Learners to Dataset Imbalance?". Learning to Learn Workshop - International Conference on Learning Representations (ICLR) [arXiv] [GitHub]
Antoniou, A., Patacchiola, M., Ochal, M., & Storkey, A. (2020). "Defining Benchmarks for Continual Few-Shot Learning". MetaLearn Workshop - Advances in Neural Information Processing Systems (NeurIPS) [arXiv] [GitHub] [YouTube] [Dataset]
Ochal, M., Patacchiola, M., Storkey, A., Vazquez, J., & Wang, S. (2021). "Few-Shot Learning with Class Imbalance". [arXiv] [GitHub]
Polvara*, R., Patacchiola*, M., Hanheide, M., & Neumann, G. (2020). "Sim-to-Real Quadrotor Landing via Sequential Deep Q-Networks and Domain Randomization". Robotics, 9(1), 8. *Co-first authors. [PDF] [GitHub]
Patacchiola, M., Fox-Roberts, P., Rosten, E. (2020). "Y-Autoencoders: Disentangling Latent Representations via Sequential Encoding". Pattern Recognition Letters, Elsevier, vol. 140, pp. 59-65. [arXiv] [DOI] [GitHub]
Thabet, M., Patacchiola, M., & Cangelosi, A. (2019). "Sample-efficient Deep Reinforcement Learning with Imaginary Rollouts for Human-Robot Interaction". IEEE/RSJ International Conference on Intelligent Robots and Systems (IROS) [arXiv]
Polvara* R., Patacchiola*, M., Sharma S., Wan J., Manning A., Sutton R., Cangelosi A. (2018). "Toward End-To-End Control for UAV Autonomous Landing Via Deep Reinforcement Learning". The 2018 International Conference on Unmanned Aircraft Systems (ICUAS). *Co-first authors. [PDF]
Surace, L., Patacchiola, M., Battini Sonmez, E., Spataro, W., & Cangelosi, A. (2017). "Emotion Recognition in the Wild using Deep Neural Networks and Bayesian Classifiers". In Proceeding of the Fifth Emotion Recognition in the Wild (EmotiW) Challenge, Glasgow, United Kingdom. [arXiv]
Patacchiola, M., & Cangelosi, A. (2017). "Head Pose Estimation in the Wild using Convolutional Neural Networks and Adaptive Gradient Methods". Pattern Recognition, Elsevier, vol. 71, pp. 132-143. [PDF] [DOI]
Patacchiola, M., & Cangelosi, A. (2016). "A Developmental Bayesian Model of Trust in Artificial Cognitive Systems". In Proceedings of the International Conference on Development and Learning and Epigenetic Robotics (ICDL-EpiRob). [PDF]
Zanatto, D., Patacchiola, M., Goslin, J., & Cangelosi, A. (2016). "Priming Anthropomorphism: Can the credibility of humanlike robots be transferred to non-humanlike robots?". In The Eleventh ACM/IEEE International Conference on Human Robot Interation (HRI). [PDF]